Super-VLOAM
Our project, Super-VLOAM, introduced a novel approach to enhance Visual-LiDAR Odometry and Mapping (VLOAM) by integrating SuperPoint and SuperGlue deep learning models. This initiative aimed to improve VLOAM’s accuracy and robustness, particularly in challenging environments like low-texture areas and during dynamic motion.
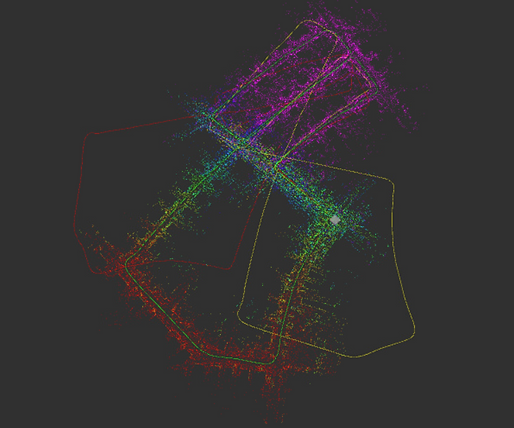
Innovation:
Super-VLOAM uniquely uses SuperPoint for self-supervised interest point detection and SuperGlue, a graph neural network, for advanced feature matching. These integrations mark a significant shift from classical feature extraction methods, offering increased robustness and interpretability.
Approach:
Our process began with replicating baseline VLOAM results. We then integrated SuperPoint and SuperGlue, initially using Pytorch models converted to ONNX format, into the VLOAM framework. Our evaluation encompassed a variety of metrics, including accuracy and computational efficiency.
Results and Analysis:
Super-VLOAM showcased promising improvements over traditional VLOAM, especially in complex scenarios. However, challenges like underperformance in certain sequences highlighted areas for further development.
Future Directions:
Our future work involves optimizing Super-VLOAM's performance on GPUs, fine-tuning deep learning models, and expanding evaluations to a broader range of scenarios to solidify its reliability and efficiency in real-world applications.
This content effectively highlights the key aspects of Aneesh's project, emphasizing the innovative approach, methodology, results, and future potential of Super-VLOAM in the field of robotics and mapping.
Read the Full Report here

